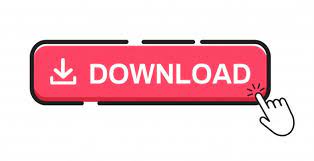
Almost every group is distinguished by whether headers such as.You can now see many things that these features have overfit to: target_names ) alt.atheism: sgi livesey atheists writes people caltech com god keith edu aphics: organization thanks files subject com image lines university edu graphics sci.space: toronto moon gov com alaska access henry nasa edu space : article writes kent people christian jesus sandvik edu com god > show_top10 ( clf, vectorizer, newsgroups_train. for i, category in enumerate ( categories ). > import numpy as np > def show_top10 ( classifier, vectorizer, categories ). When using these images, please give credit to AT&T Laboratories Cambridge. The original dataset consisted of 92 x 112, while the version available here Relatively small dataset is more interesting from an unsupervised or Identity of the person pictured however, with only 10 examples per class, this The “target” for this database is an integer from 0 to 39 indicating the Which are easier to work with for many algorithms. The loader will convert these to floating point values on the interval , The image is quantized to 256 grey levels and stored as unsigned 8-bit integers Homogeneous background with the subjects in an upright, frontal position (with Subjects, the images were taken at different times, varying the lighting,įacial expressions (open / closed eyes, smiling / not smiling) and facialĭetails (glasses / no glasses). There are ten different images of each of 40 distinct subjects. produces Gaussianĭata with a spherical decision boundary for binary classification. centroid-basedĬlustering or linear classification), including optional Gaussian noise. Make_circles and make_moons generate 2d binary classificationĭatasets that are challenging to certain algorithms (e.g. Make_hastie_10_2 generates a similar binary, 10-dimensional problem. Near-equal-size classes separated by concentric hyperspheres. Make_gaussian_quantiles divides a single Gaussian cluster into Per class and linear transformations of the feature space. Make_classification specialises in introducing noise by way of:Ĭorrelated, redundant and uninformative features multiple Gaussian clusters Standard deviations of each cluster, and is used to demonstrate clustering. make_blobs provides greater control regarding the centers and Both make_blobs and make_classification create multiclassĭatasets by allocating each class one or more normally-distributed clusters of
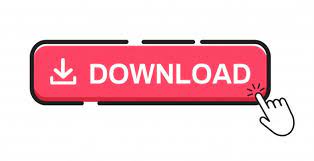